Overloading has a significant impact on the condition of a vehicle, for example, it can cause the tires to overheat and wear rapidly or lead to cracks in the vehicle chassis. The premature wear and tear resulted from frequent overload might also cause the vehicle warranty to become void, since driving overloaded can be prevented by the vehicle operators.
In order to support the military vehicle operators we have developed a HUMS based Load indication solution, that can detect overload automatically and signal to the driver that adjustments have to be made in order to guarantee a correct usage of the vehicle.
To demonstrate the ILIAS I-HUMS® capabilities on detecting vehicle overload, we have verified two methodologies: mechanical and machine learning. Both methodologies have been tested on FOX tactical vehicles in the environment of Belgian Defense, by using the following vehicle measurements:
- X-, Y- and Z-axis accelerometer
- Pitch, yaw and roll (gyroscope)
- CAN-bus data (among which engine load, intake manifold absolute pressure, mass air flow rate)
Reference drives have been performed in early March 2020 where the Belgian Defense FOX vehicles were driven by different drivers on routes incorporating on-road and off-road terrain, normal roads and highways, and characteristics that allowed accelerating, braking and turns. This type of reference drives were necessary in order to eliminate the driver behavior as factor influencing the load estimation.
MECHANICAL MODEL
For the mechanical model we are collaborating with the Department of Mechanical Engineering at the Technical University of Denmark, where we have tested a vehicle mass estimation methodology based on the data captured with the ILIAS I-HUMS®.
The initial results are based on a Load indication model based on the following constants:
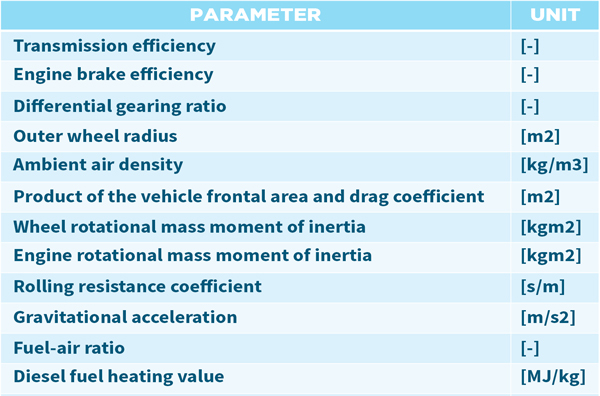
Results
The following results have been obtained when analyzing the data coming from armored FOX vehicles, with the vehicles being both loaded and unloaded:
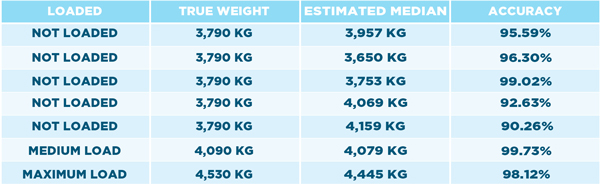
The results from the mechanical model show an increasing accuracy in determining vehicle load in the cases where the vehicles had medium and maximum loads. This represents a good foundation for the model since our aim is to indicate to the driver the overload situations that have the most negative impact on vehicle health.
This approach will be further enriched with a machine learning-based algorithm.
MACHINE LEARNING MODEL
Initial results have been accomplished in collaboration with Sirris, our data science partners, based on a data-driven methodology for the detection of an overloaded vehicle. The achieved load indication milestone represents one of the use cases for our joint project STUNT, which targets diSTributed product Usage aNalyTics in the context of asset management of off-road and special-purpose vehicles in mobile operations.
With the machine learning load indication model the aim was to use the ILIAS HUMS® data and develop an algorithm that assigns a label to each drive of a vehicle, indicating whether that drive was executed under normal load, low overload, medium overload or high overload. In order to recommend an action to the driver as fast as possible, we want to classify the drive already within a few seconds after it starts.
The FOX vehicle data was derived into features with the purpose to capture the vehicle dynamics while driving. These features are needed so that the machine learning algorithm can use them to distinguish between the different overload situations.
The load indication algorithm uses the following features over a rolling window of 10 seconds:
- Average and standard deviation of engine speed
- Vehicle acceleration, maximum, minimum and average speed
- X-, Y- and Z-axis accelerometer
- Average and standard deviation of engine load
- Average and standard deviation of throttle position
- Average and standard deviation of intake manifold absolute pressure
Results
A Random Forest classifier was identified as the most performant and consistent classification algorithm, leading to the following results, where on the x- axis we find the predicted class by the algorithm, while on the y-axis, we find the actual class.
The machine learning algorithm has identified the following results:
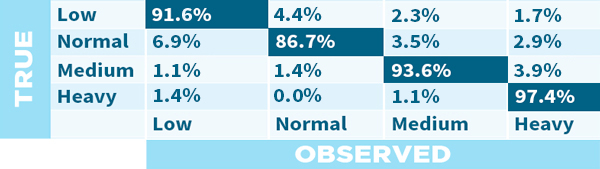
As it can be observed in the table, the results emphasized in blue support the initial conclusions from the mechanical model, where the higher the vehicle load, the more accurate the model becomes.
CONCLUSIONS AND WAY AHEAD
The analysis provided in this article demonstrates that the right approach has been taken and the high accuracy for the initial results supports that. The mechanical and machine learning models have provided similar results which indicates that using them together will increase the capabilities of the ILIAS I-HUMS® in estimating vehicle overload.
With this initial steps we have demonstrated the capability of the ILIAS I-HUMS® product as a reliable system that supports the driver take action to avoid premature tear and wear of the vehicle.
The approach described here validates the flexibility of our system where it can be easily adapted to provide overload information coming from different types of military vehicles.
If you would like to find out more about the overload indication project setup, please do not hesitate to contact us.