
CASE STUDY: DETECTING USAGE ON A COMPACT EXCAVATOR WITH ILIAS NVO TECHNOLOGY
Following our acquisition of NVO (Northern Vehicle Operations), the capability of the ILIAS Defense Platform has significantly increased in the domain of HUMS and Predictive Fleet Management. Thanks to the I-TLS® and I-HUMS® products we can provide smarter solutions for the support of Performance Based Logistics through Intelligent Health and Usage Monitoring Systems. Moreover, ILIAS NVO opens up exciting new prospects for advances in machine learning and predictive maintenance.
This article describes an ILIAS NVO case study where we detect usage on a compact excavator to illustrate the art of the possible of this new capability.
Compact excavators are often rented on an hourly or daily rate. No counters are used, which means that for billing only calendar hours or days are used. For maintenance, the system has an “engine hour” counter, but this gives indicator only when the system is running (idle or driving or operating).
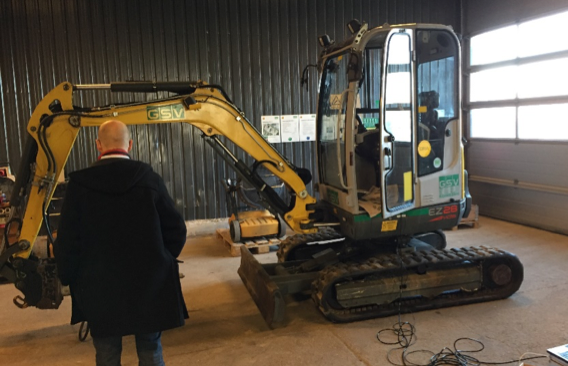
We wanted to introduce other counters for more precise information on the actual use of the machine. One Inertial Measurement Unit (IMU) sensor provides a very cheap way of getting much more usage data.
Business case
For the rental case, a “power by the hour” rate could be more efficient. I.e. the end customer pays for the required usage or wear of the machine and not just for the number of hours it is reserved. This would give a fairer pricing model, since the real cost of running the machine is mostly due to maintenance. This would also give the user an incentive to take care of the machine while using it. It gives a better way to estimate the need for maintenance or to balance out the usage of equipment.
For other cases, a simple ILIAS NVO IMU sensor could give benefits of getting higher fleet availability, lowering operating costs etc. by doing the following:
- Monitor machine health and how to predict asset failure (predictive maintenance)
- Prevent or detect abuse
- Provide data for warranty models
- Provide data for fleet management/optimization
All of the above mentioned points can be addressed with a simple and robust IMU.
Proof of concept
For this proof of concept, we wanted to test the data collection and analytic capability of such a system:
“We believe that we can measure how many hours a hammer and tracks / undercarriage have been used on a compact excavator by measuring the vibration pattern”
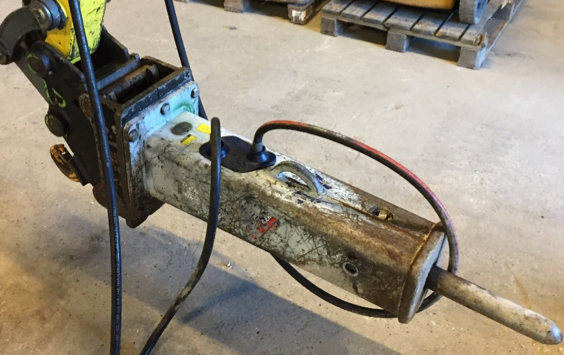
As proof of concept we wanted to be able to detect the following states:
- Engine Off – ID 4001
- Idle – low RPM ID 4002
- Idle – High RPM ID 4003
- Driving – Turtle gear ID 4010
- Driving – Rabbit gear ID 4011
- Driving – Slalom ID 4012
- Hammer – ID 4020
Other states (such as abuse or hard usage) could also be detected.
The Machine Learning approach
A single IMU sensor was installed in the frame of the vehicle. Data was collected with high resolution and high sampling frequency. Data was collected on a small embedded device in the vehicle.
Model creation data
A series of tests with before mentioned states were made. The data was labeled with each state. After data labeling, a decision tree was created using statistical features of the data. The decision tree could then be applied to data collected in real time, on the embedded device.
Test results
A new series of tests were made. This data was again labeled with each state. Data was collected and parsed with the decision tree generated with the model data from before (with fixed data chunk sizes). In the figure below, the results from the algorithms can be seen.

On the top row of bars, the data labels (the truth) are shown, colored. In the next row of bars, the detected states are colored. The bottom graph is a visualization of part of the collected raw data from the ILIAS NVO IMU.
As seen, the colors match with very high precision. Only in the beginning and end of the states there are small errors. This is most likely because of the data labeling (i.e. as the labels were created manually with a stopwatch they may not be completely timely)
Test conclusion
The IMU sensors and embedded device mounted on the Compact Excavator are able to provide data for machine learning and recognition of at least 6 different usage patterns:
- ignition
- idle
- slow driving
- fast driving
- slalom driving
- hammering
This usage information can now be collected, and can support a “power by the hour” agreement between the operator and the OEM or service provider. The operator can request for capacity, and the OEM or service provider can follow-up on actual consumption of this capacity for maintenance and billing purposes.
The whole setup was made within a few hours. Mounting of the system took thirty minutes, calibration and collecting model creation data took an hour. Creating the models took thirty minutes. Testing and validating the system took another hour.
Perspective
This ILIAS NVO IMU sensor and embedded system provide a very easy way of capturing actual and valid usage information on mechanical systems. It can easily detect multiple states. The counters provided could also be summarized and be used to provide the operator with information on when it is time to replace the hammer – before it actually breaks. The time saving from this alone are enough to pay for the system.
If you want to know more about ILIAS NVO do not hesitate to ask for a demonstration: